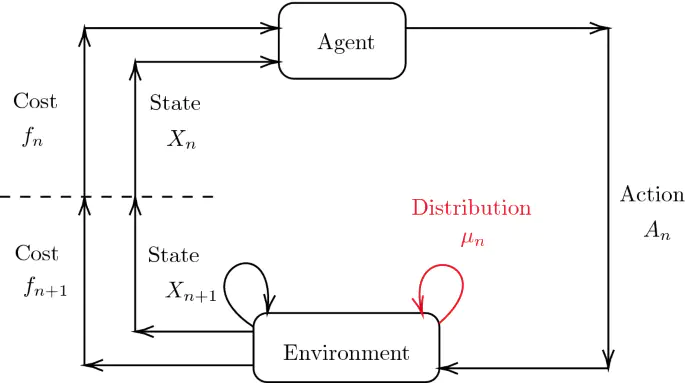
In this project we consider online reinforcement learning in Multi-agent setting using the Mean-Field Game paradigm. Unlike traditional approaches, we alleviate the need for a mean-field oracle by developing an algorithm that estimates the mean-field and the optimal policy using the single sample path of the generic agent. We call this {\it Sandbox Learning}, as it can be used as a warm-start for any agent operating in a multi-agent non-cooperative setting. We adopt a two timescale approach in which an online fixed-point recursion for the mean-field operates on a slower timescale and in tandem with a control policy update on a faster timescale for the generic agent. Given that the underlying Markov Decision Process (MDP) of the agent is communicating, we provide finite sample convergence guarantees in terms of convergence of the mean-field and control policy to the mean-field equilibrium. The sample complexity of the Sandbox learning algorithm is $\mathcal{O}(\epsilon^{-4})$. Finally, we empirically demonstrate the effectiveness of the sandbox learning algorithm in diverse scenarios, including those where the MDP does not necessarily have a single communicating class.